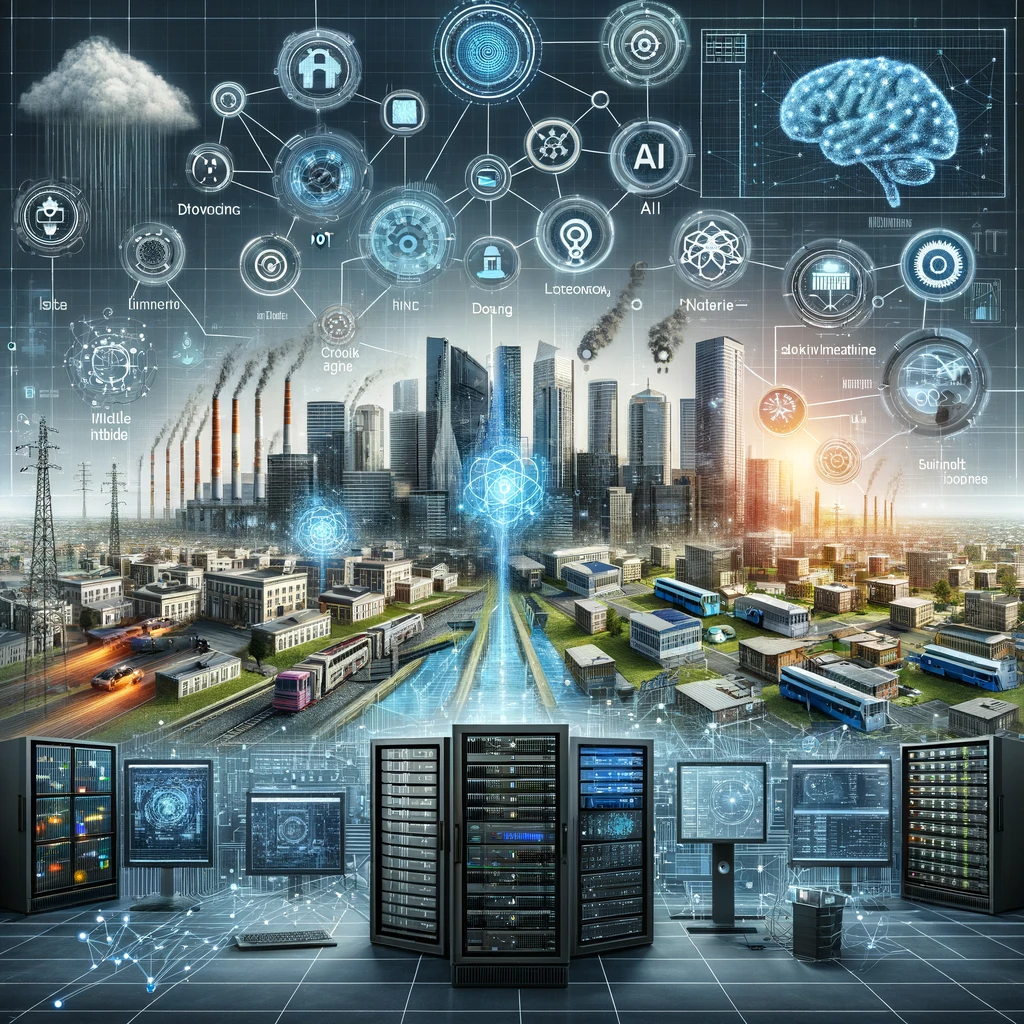
Digital twin technology, an intriguing blend of virtual and physical worlds, relies on a robust foundation of software and hardware components. This sophisticated technology integrates Internet of Things (IoT), artificial intelligence (AI), machine learning, and data analytics, creating dynamic virtual models that mirror physical entities in real-time. These models provide invaluable insights and predictive capabilities across various industries, from manufacturing to urban planning.
The core of digital twin technology starts with the IoT. IoT devices are equipped with sensors and actuaries that gather data from their physical surroundings, which could include everything from temperature and pressure in industrial machines to traffic flow and energy usage in smart cities. These devices are essential for collecting the vast amounts of real-time data necessary for creating accurate and actionable digital twins.
AI and machine learning are pivotal in interpreting this data, allowing digital twins to not only replicate physical objects but also predict their future states and respond to changes proactively. AI algorithms help in processing and analyzing the data to detect patterns, anomalies, and trends. Machine learning takes this a step further by enabling the digital twin to learn from data over time, continuously improving its accuracy and functionality based on feedback loops from the physical environment.
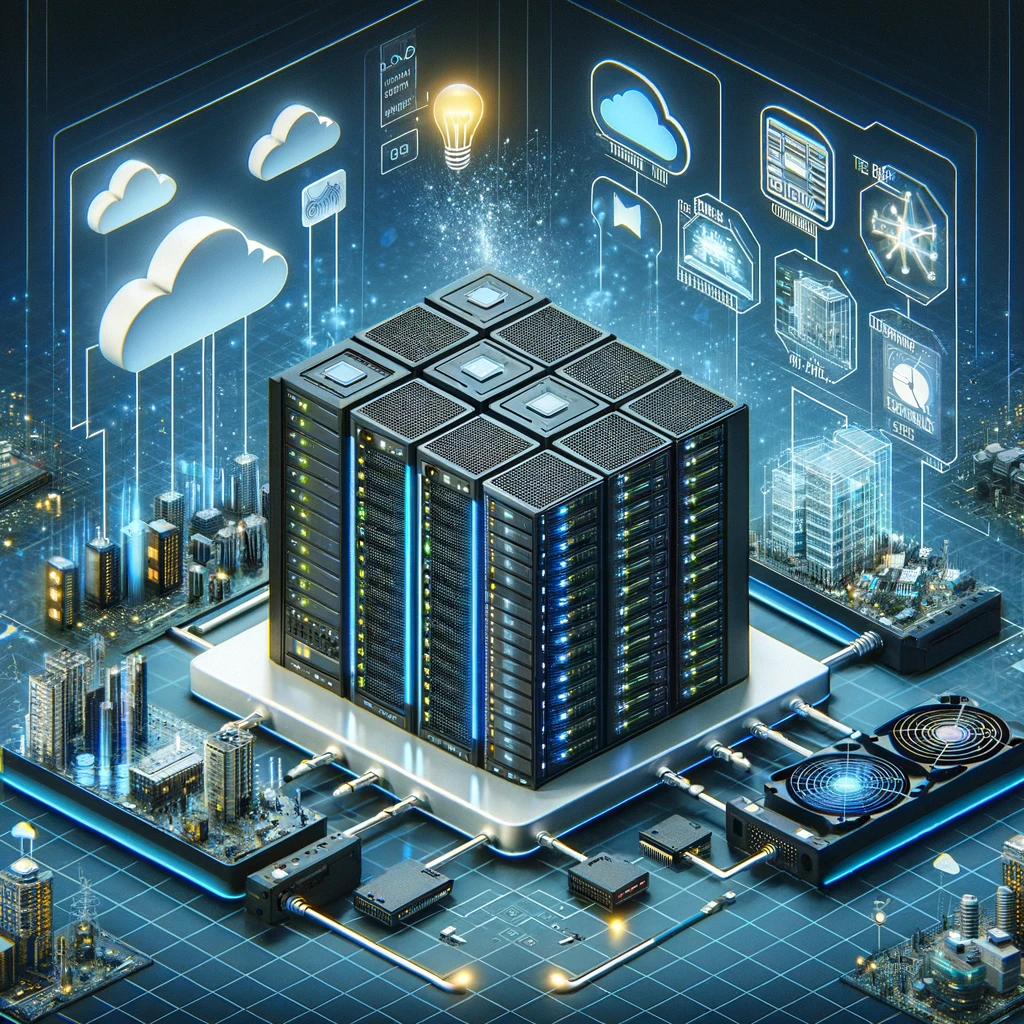
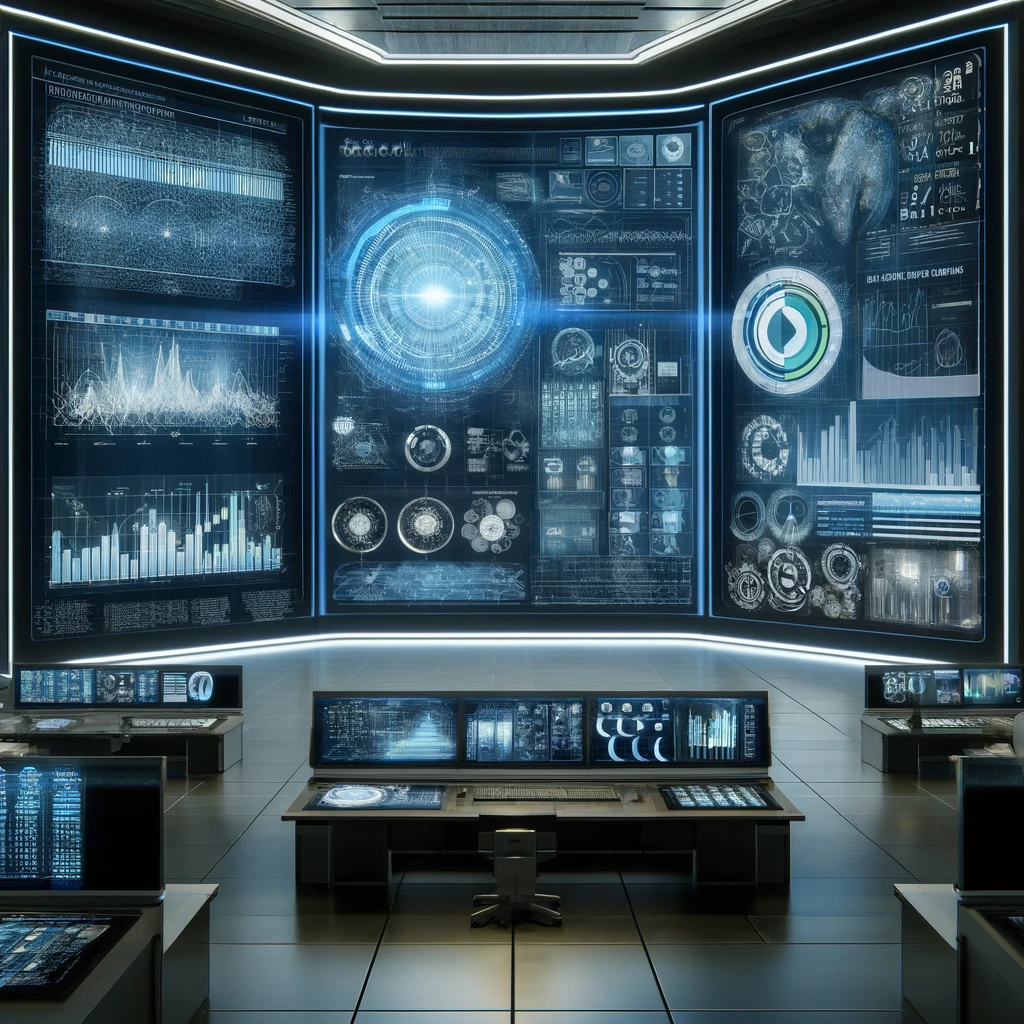
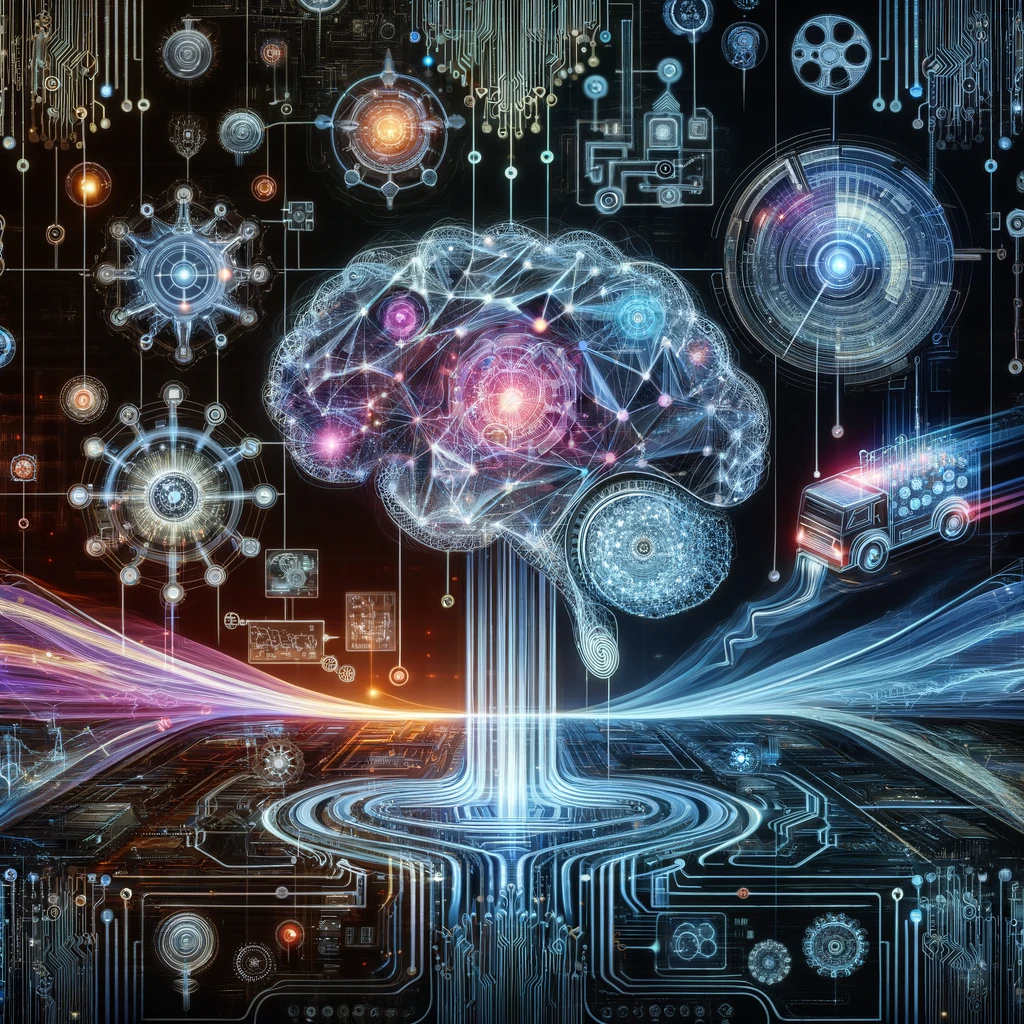
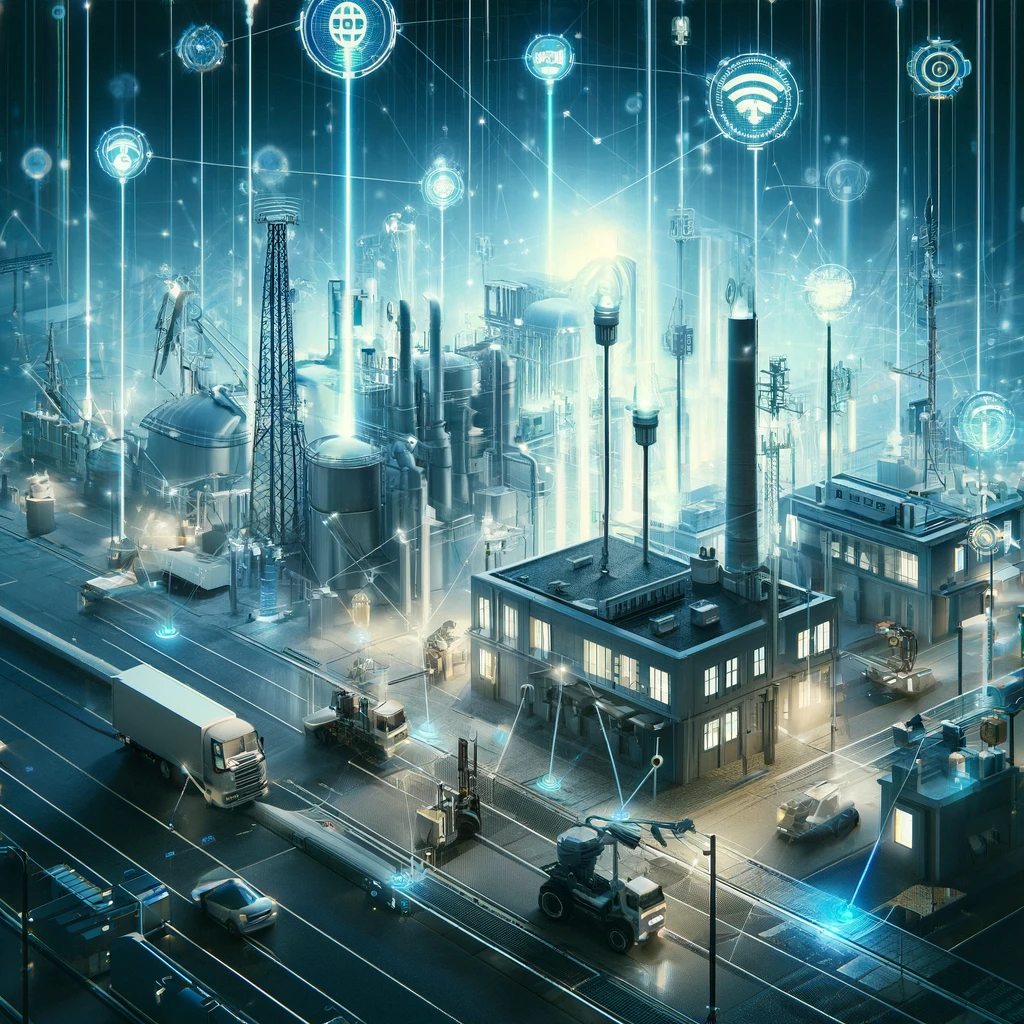
Data analytics is another crucial component, turning raw data into meaningful insights. Advanced analytics techniques are applied to interpret the data collected, which can be used for various purposes such as predictive maintenance, optimization processes, and innovation in product design. These analytics are supported by robust data management systems that ensure data integrity and security.
At the heart of a digital twin is the simulation software that creates and manages the virtual models. This software is highly complex, often relying on 3D modeling tools and advanced simulation algorithms that can accurately represent physical objects in a virtual space. Furthermore, the software must integrate seamlessly with AI and analytics platforms to allow for real-time data processing and simulation adjustments based on incoming data.
The software architecture typically involves several layers including data ingestion, data storage, data processing, and user interface layers. The data ingestion layer handles the input from various IoT devices, while the storage layer organizes this data efficiently. The processing layer applies machine learning and analytics to interpret the data, and the user interface layer allows users to interact with, visualize, and manipulate the digital twin.
The hardware required for digital twins must be capable of supporting extensive data collection, processing, and simulation tasks. This usually involves IoT sensors and devices that are robust enough to operate under different environmental conditions and can transmit data securely and efficiently.
The network infrastructure must be reliable and fast, as digital twins require high bandwidth to process and analyze data streams in real-time. Edge computing devices also play a role in handling data processing locally at the edge of the network, reducing latency and improving response times in critical applications.
Cloud computing platforms are increasingly used to scale the processing and storage capabilities needed for digital twins. These platforms provide the elastic scalability required for handling large-scale digital twin implementations, offering powerful computational resources and storage options.
The technology behind digital twins is a sophisticated amalgamation of IoT, AI, machine learning, and data analytics, underpinned by powerful software and hardware components. As these technologies continue to evolve, the functionality and applications of digital twins are expected to expand, driving further innovation in the way we interact with and understand our physical world. The integration of these technologies ensures that digital twins are not just reactive systems but proactive ones that enhance decision-making and operational efficiency.